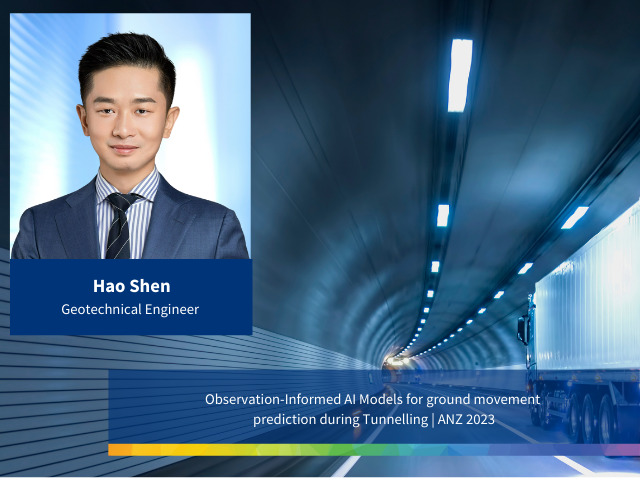
Tetra Tech Coffey’s Geotechnical Engineer, Hao Shen presented a paper at the 14th Australia and New Zealand Conference on Geomechanics in Cairns, Queensland (ANZ2023). Co-written with Geoservices Leader, Ignacio Ortega, the paper is titled “Predicting Tunnelling-Induced Ground Movement Using an AI Cloud App”.
Utilising a cloud-based AI application built on the VIKTOR platform, Hao and Andreas have been able to lead the development of this cutting-edge application to enhance the future of tunnelling predictions. Here Hao explains more.
Tell us what drove the need for your research and APP development?
In urban metro tunnel construction, there’s an influx of available data. Among all types, ground settlement monitoring records are the most readily accessible, offering a golden opportunity for AI algorithms to extract valuable insights into ground responses due to tunnelling.
As Geotechnical engineer specialists, we are supporting a number of clients on major tunnelling work, and we have seen the opportunity to rethink our approach and enhance our design and risk management strategy.
What is some of the background to your technical approach?
Typically, predictions of these tunnelling-induced ground responses leaned heavily on empirical formulations (Peck 1996), analytical solutions (Loganathan, N., Poulos, H.G. and Xu, K.J. 2001), or advanced numerical modellings. The problem with empirical formulations is that it requires the determination of tunnelling-induced volume loss in the first place. And while analytical solutions had their value, they were often restricted by certain boundary conditions, ground materials, and assumptions made during their creation.
Finally, incorporating “raw” Shield Operational Data along the tunnel alignment presents a significant challenge for any numerical modelling method. In an Earth Pressure Balanced (EPB) shield tunnelling project, this data usually comprises Torque, Thrust, Penetration rate, Face pressure, and Rotation speed.
Long Short-Term Memory (LSTM) proposed by (Schmidhuber, J., 1997) is a specialised type of Recurrent Neural Network (RNN), which is particularly effective when dealing with sequential data. In the context of predicting sequential ground movements in tunnelling, LSTM networks shine due to their ability to remember and learn from long-term dependencies in the data.
This feature makes LSTM ideal for recognising patterns over time, allowing for more accurate predictions of future ground movements based on historical data. This can provide valuable insights and predictions for tunnelling projects, leading to safer and more efficient operations. With the published data from (Pin Zhang et. al. 2020), I have thus trained an LSTM model.
How have you built AI into your model?
What has really excited me about this advancement has been the ability to take it a step further to develop a cloud application built via the VIKTOR platform. The app we have developed allows engineers to forecast tunnelling-induced ground movement, in terms of volume loss and settlement profiles. What’s more, it can be tailored to fit the specific requirements by tweaking the geometrical, shield operational, and geotechnical parameters.
At the centre of the application is the AI model that employs Long Short-Term Memory (LSTM) neural networks, bringing tunnelling predictions at your fingertips.
The Cloud APP is available now via the VIKTOR application development platform.
Connect with Hao at hao.shen@tetratech.com
Disclaimer of the author: While this application is a powerful tool designed to provide insights and forecasts based on the data inputted, it is intended for illustrative and informational purposes only, as the LSTM model was trained only based on published data.
It should not be used as the sole tool for decision-making in real-life tunnelling projects or operations. The creator of this application cannot be held liable for any decisions, actions, or outcomes resulting from its use.